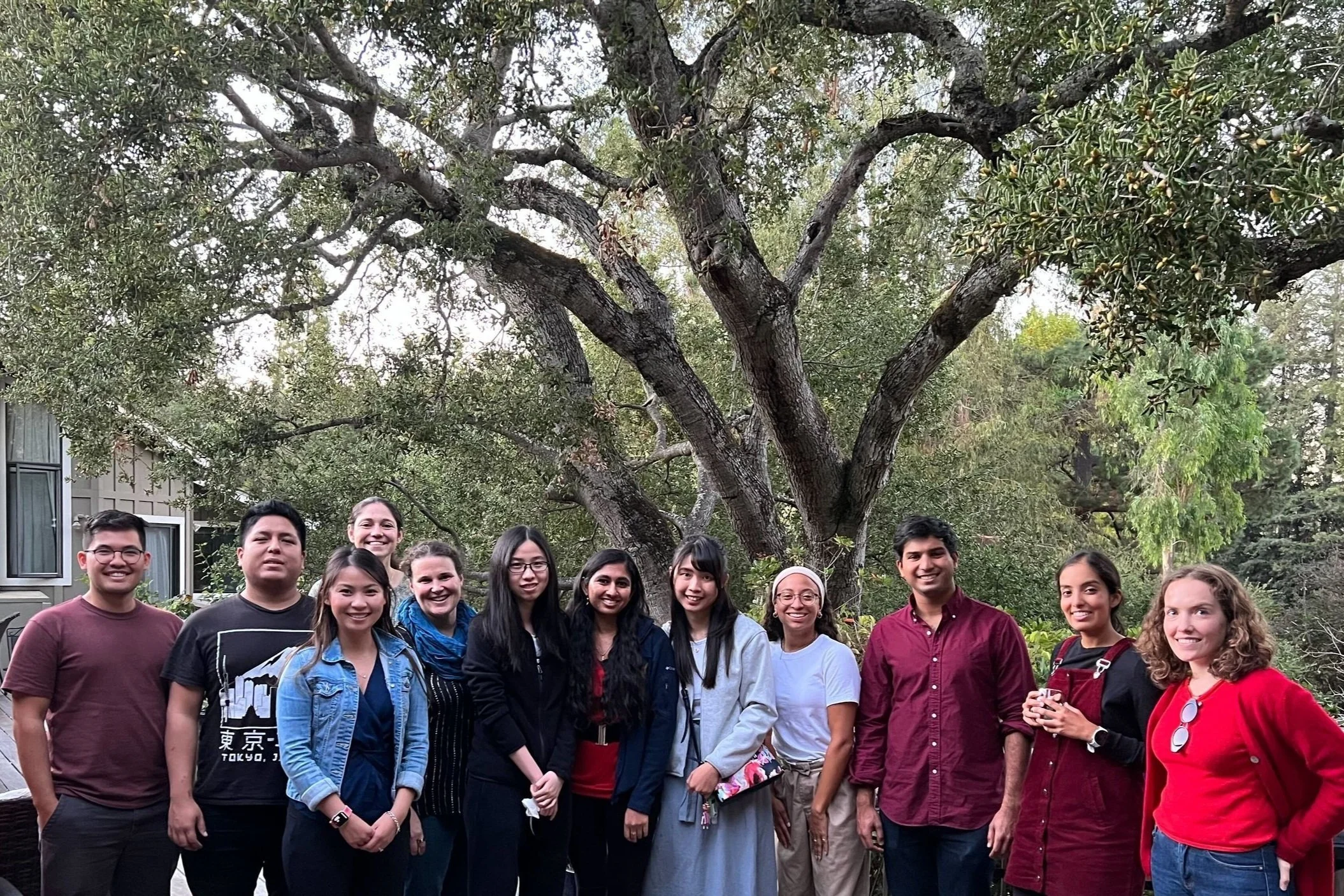
Engelhardt Research Group @ Gladstone Institutes and Stanford University
-
We model state.
From the behavior of single cells to clinicians’ treatment objectives to regions of interest in spatial genomics data to single-cell expression QTLs, we built statistical models that capture relevant state in biomedical data. Many of our models are built on structured dimension reduction approaches, carefully modeling the data likelihood and known biological structure.
-
We predict intervention effects.
How will a patient with end-stage renal disease respond to electrolytes? How will T cells change their behavior with CRISPR perturbations? How does cell cycle change in brains of individuals with early-life trauma? How do CRISPR edits affect the relationships between pairs of genes? We build predictive models to capture these dynamics.
-
We design experiments.
With these models in hand, we can return to biologists and clinicians methods for decision making. We select and prioritize follow-up experiments including interventions, CRISPR modifications, protein edits, tissue slices, or small molecules. In the clinic, our reinforcement learning methods work as clinician-in-the-loop decision support tools for electrolyte repletion, lab testing, ventilator weaning, and more.